COMPAS, a piece of software commonly used in the justice system to predict which offenders will find themselves behind bars again, is no better than soliciting random people on Mechanical Turk to determine the same thing, a new study has found. Oh, and they’re both racially biased.
Julia Dressel and Hany Farid of Dartmouth college looked into the system amid growing skepticism that automated systems like COMPAS (Correctional Offender Management Profiling for Alternative Sanctions) can accurately predict something as complex as recidivism rates.
To test this, they recruited people on Amazon’s Mechanical Turk to review an offender’s sex, age and criminal record (minus, of course, whether the person did eventually recidivate, or reoffend). The people were then asked to provide a positive (will recidivate) or negative (will not recidivate) prediction; evaluations of the same offender were pooled and the prediction determined by majority rule. The same offenders were also processed in COMPAS’s recidivism prediction engine.
As it turns out, the untrained humans and the complex, expensive software achieved nearly the same exact level of accuracy — low, to be precise. Humans correctly predicted reoffenders about 67 percent of the time, while COMPAS got it about 65 percent of the time. And the two groups only agreed on about 70 percent of the offenders.
Now, if the point of this software was to correctly replicate unskilled randos being paid next to nothing to do something they’ve never done before, it almost succeeds. That doesn’t seem to be the case.
In fact, the researchers also found that they could replicate the success rate of COMPAS by only using two data points: age and number of previous convictions.
“Claims that secretive and seemingly sophisticated data tools are more accurate and fair than humans are simply not supported by our research findings,” said Dressel. “The use of such software may be doing nothing to help people who could be denied a second chance by black-box algorithms.”
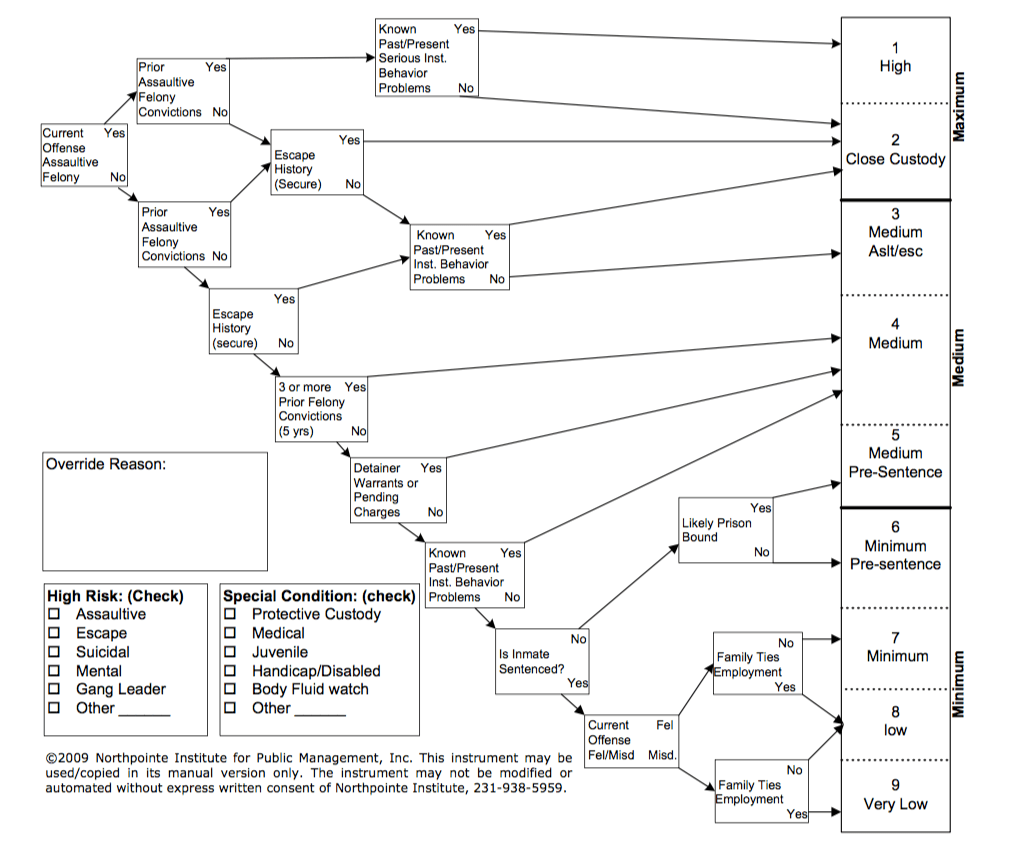
Example of a manual decision tree for classifying offenders.
As if all that wasn’t enough, it was further found that both the human groups and the COMPAS classifier show a rather mysterious form of racial bias.
Both tended toward false positives for black people (i.e. they were predicted to reoffend but in reality did not) and false negatives for white people (vice versa). Yet this bias appeared whether or not race was included in the data by which offenders were evaluated.
Black offenders do have higher recidivism rates than white offenders in the data set used (for reasons too numerous and complex to get into here), but the evaluations don’t reflect that. Black people, regardless of whether the evaluators knew their race, were predicted to reoffend more than they did, and white people predicted to reoffend less than they did. Given that this data may be used to determine which offenders receive especial police attention, it may be that these biases are self-perpetuating. Yet it’s still unclear what metrics are operating as surrogate race indicators.
Unfortunately the question of fairness must remain unanswered for now, as this study was not geared toward finding an answer to it, just to finding the overall accuracy of the system. And now that we know that accuracy is remarkably low, one may consider all COMPAS’s predictions questionable, not just those likely to be biased one way or another.
Even that, however, is not necessarily new: a 2015 study looked at nine such automated predictors of recidivism and found that eight of them were inaccurate.
Equivant, the company that makes COMPAS, issued an official response to the study. Only six factors, it writes, are actually used in the recidivism prediction, not the 137 mentioned in the study (those data are used for other determinations; the software does more than this one task). And a 70 percent accuracy rate (which it almost reached) is good enough by some standards, it argues. In that case, perhaps those standards should be revisited!
It’s not for nothing that cities like New York are implementing official programs to look into algorithmic bias in systems like this, whether they’re for predicting crimes, identifying repeat offenders or convicting suspects. Independent reviews of these often private and proprietary systems, like the one published today, are an essential step in keeping the companies honest and their products effective — if they ever were.
source:-tc